Shear-Wave Elastography Assist
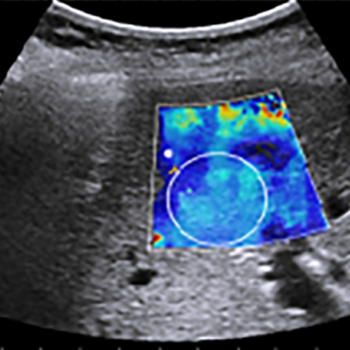
In collaboration with physicians at Mass General Brigham, researchers at Lincoln Laboratory developed this AI-based software tool to aid physicians with analysis of shear-wave elastography (SWE) and ultrasound. SWE-Assist provides a highly reliable classification of liver-fibrosis staging indicative of disease. This capability could lead to accepted clinical use of ultrasound SWE in diagnosing and monitoring liver diseases.
Motivation
Researchers reported in the journal Hepatology that, as of 2016, approximately 24% of the world's population has nonalcoholic fatty liver disease (NAFLD), with an estimated 64 million people in the United States being afflicted. They also report that NAFLD is underdiagnosed, primarily because symptoms are not recognized early, and it has also been reported that no routine screening for the disease is yet cost-effective and noninvasive. Untreated, NAFLD ultimately culminates in cirrhosis, a state of severe and irreversible liver fibrosis that might only be mitigated by a liver transplant. In the United States, NAFLD accounts for annual direct medical costs of about $103 billion.
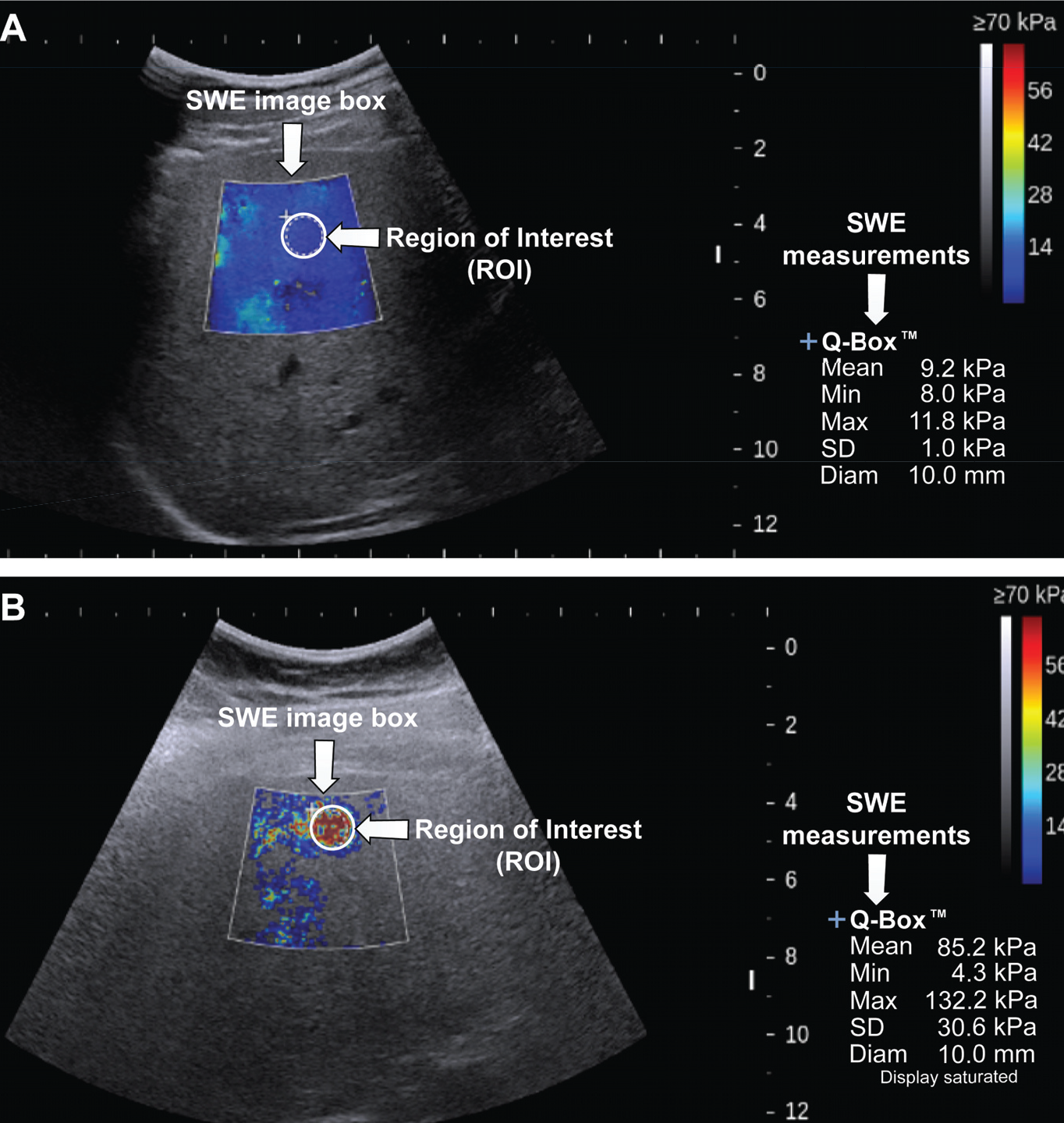
Shown are example SWE images for liver fibrosis staging. In A is a high-quality SWE image with good color fill-ins that allow measurement of liver tissue stiffness to evaluate the potential for fibrosis. In B is a low-quality image ineffective for evaluation.
Potential for Early Diagnosis
Researchers at Lincoln Laboratory and Mass General Brigham are exploring a new approach to screening for NAFLD. Their approach offers the potential for widespread early detection of, and thus early interventions for, the disease. They have developed an artificial-intelligence (AI)-based software, SWE-Assist, to analyze ultrasound SWE images and to perform liver fibrosis staging. Ultrasound SWE images showing tissue stiffness above a certain stage can be used as an indication of NAFLD; however, analyzing these images requires an operator to manually scan and assess roughly ten images to make a determination about the anomalous tissue. Manual analysis also suffers from high intra- and inter-observer variability. To address these challenges, SWE-Assist uses a deep learning−based approach to analyze just four images on which it performs three sequential functions:
- Evaluation of a SWE image to determine whether it is of sufficient quality to process
- For a quality image, selection of a homogeneous region of interest (ROI) that avoids confounders such as blood vessels and lesions
- Classification of liver fibrosis above a threshold level via a machine learning algorithm
The SWE-Assist workflow begins with a conventional shear-wave elastogram available from a Digital Imaging and Communications in Medicine (DICOM) database. SWE-Assist then determines the quality of that image and, if the image has sufficient color fill-ins, automatically selects the region of interest to perform liver fibrosis staging. The SWE-Assist algorithm extracts the relevant features of the tissue from qualified SWE images and correlates them with known pathology of liver fibrosis related to NAFLD.
Benefits
- A noninvasive and reliable diagnostic and screening alternative to liver biopsy, avoiding an invasive, expensive procedure that only targets a fraction of liver
- Potential for widespread, routing screening and treatment tracking because of its high portability and low cost
- More objective, consistent liver-fibrosis staging than achieved via human manual evaluation, which suffers from high variability
- Improved clinical flow and shortened screening process, enabled by the need for fewer than half the typical number of SWE image acquisitions for evaluation
Potential Use Cases
- Early diagnosis of liver disease
Additional Resources
Patent application 20210022715
L.J. Brattain et al., "Objective Liver Fibrosis Estimation from Shear Wave Elastography," Proceedings of the 40th Annual International Conference of the IEEE Engineering in Medicine and Biology Society, July 2018.