Standoff Detection of Concealed Threats

Detection of concealed threats is critical for protecting mass transit stations and other difficult-to-secure environments. HIVE (Hierarchical Inference for Volumetric Estimation), a custom neural network architecture, interprets volumetric imagery from standoff, active millimeter-wave scanners and automatically detects threats on people or in bags. The software enables security personnel to protect people and infrastructure in crowded environments where traditional security screening is difficult and time-consuming to implement.
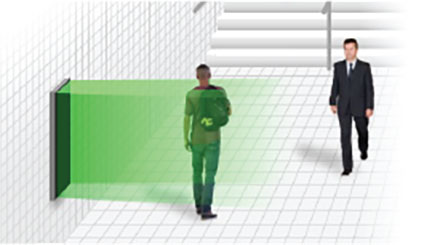
Challenge
In crowded public venues, such as mass-transportation stations, shopping centers, or sports arenas, the volume of foot traffic makes it impossible for security personnel to manually screen every person and inspect every bag. When body scanners or other microwave imagers are used for screening, the imagery is difficult for security personnel to interpret and creates concerns about customer privacy.
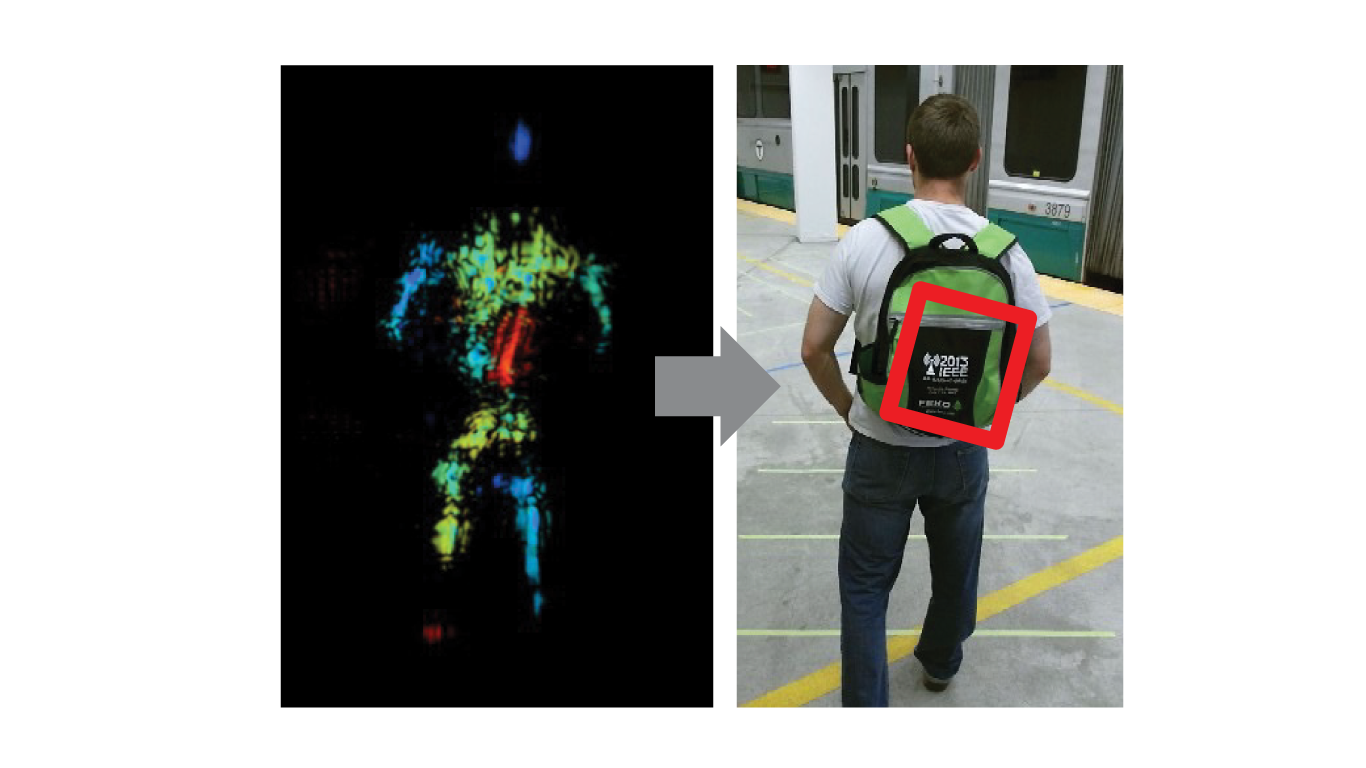
Solution
Lincoln Laboratory's novel object-detection algorithm, HIVE, automates information extraction from video-rate millimeter-wave imagery that shows the reflection of microwave radiation on objects. The algorithm selects the portions of an image that may contain a man-made object, estimates a bounding box around the detected object, and homes in to classify the object as one of several specified threat classes. The analytics system then uses all this information to automatically generate threat alerts and visualization products for use by security personnel. The HIVE algorithm pioneers the use of deep convolutional neural networks to process the high-fidelity, complex-valued, 3D volumetric imagery. Within HIVE, data are subsequently transformed into a 2D representation to reduce processing load and leverage common machine learning techniques to train the algorithm. The 2D output also allows the system to generate easy-to-understand visualizations. Most importantly, because HIVE provides more information to security personnel than existing systems can, it enables insight into the nature of the threat object, its location in the scene, and the reason it was detected.
Benefits
- HIVE operates on video data acquired by a new class of active RF sensors that unobtrusively screen people while they are in motion, instead of requiring them to stop, pose, or remove belongings
- Machine learning automation saves time and resources of security personnel and is more reliable than human interpretation of RF imagery
- HIVE can be trained for different purposes, e.g., detecting particular objects or recognizing specific materials like metals
- A visualization showing just the objects carried in a bag protects a subject's privacy because security personnel do not see imagery of the person's body
- The algorithm can be paired with different active RF systems to add new capabilities and improved detection performance as commercial imaging technology advances